- Programmes in English 2025/2026
- Admission 2025/2026 Scholarships
- For exchange students
- Free Movers
- Transfer studies
- Erasmus+ studies and traineeships
- Mentor programme
- Student testimonials
- Accommodation
- Career Services
- Medical Care
- Immigration Regulations
- Leisure and Student Activities
- Useful information
- VILNIUS TECH for Creators of Tomorrow
- Mental and spiritual support
- Representatives Abroad
- Contacts
- Computer Engineering
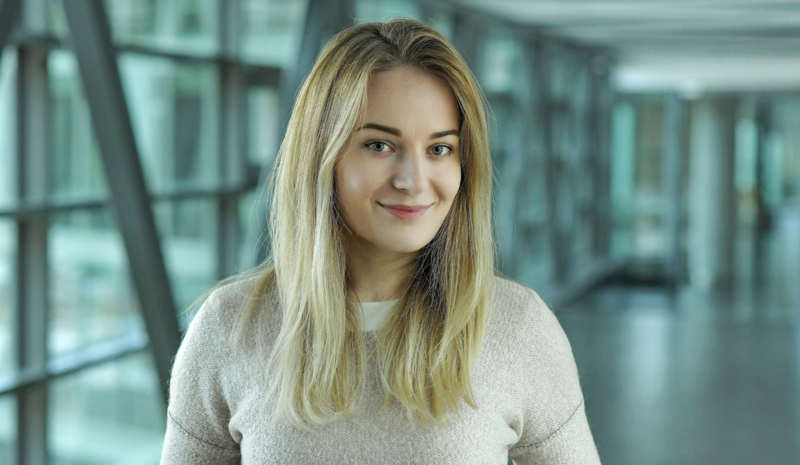.png)
2024-02-05
How will artificial intelligence help save lives?
Lung diseases are among the most impactful on the human body and quality of life. Therefore, early diagnosis is a crucial factor for recovery. However, even with the most advanced medical techniques, timely diagnosis of lung cancer, tuberculosis, or the effects of diseases caused by COVID-19 does not always happen.
Edita Mazoniene, a doctoral student at Vilnius Gediminas Technical University (VILNIUS TECH) Faculty of Fundamental Sciences, explores how artificial intelligence can assist in identifying the consequences of lung diseases in the early stages of diagnosis.
Treatment for lung diseases costs 380 billion euros annually
Over the past three decades, researchers when analysing various indicators related to chronic respiratory diseases and patient data have found that the prevalence of various lung diseases has increased significantly due to population growth, environmental hazards, and longer life expectancy. It has also been estimated that 4 million people worldwide die from lung diseases, and European Union (EU) countries spend about 380 billion euros on their treatment. Therefore, early diagnosis and accurate classification of the disease, detecting early changes, and assessing the cause rather than the consequence, could gave a great impact on progression of lung diseases globally.
According to E. Mazoniene, most cases of lung diseases go unnoticed. For example, tuberculosis in its early stages is often confused with other diseases, resulting in patients not receiving timely and appropriate treatment, further complicating the condition and progression of the disease.
For faster and more accurate diagnosis of such diseases, E. Mazoniene employs artificial intelligence in her research, specifically convolutional neural networks used for image recognition and object identification in X-ray image analysis.
"Convolutional neural networks can be trained to compare and properly classify radiological images by applying a deep convolutional neural network model that automatically identifies, for example, cases of tuberculosis. After multiple experiments, researchers have achieved an accuracy of up to 98.6%," explains E. Mazoniene.
These networks can also be used for lung segmentation, playing a crucial role in computerized diagnostics. This is because the entire set of acquired images is transformed into a dataset and then analysed internally, layer by layer, rather than visually. This approach provides reliable indicators, unlike decisions based on X-ray interpretations, where changes or forming structures are not always clearly visible, which then gets analysed by a radiologist. Therefore, such data analysis can become a suitable biomedical tool for early lung disease diagnostics, providing significant assistance to doctors in differentiating diseases or identifying early disease.
It will also help in the fight against COVID-19
E. Mazoniene explains that as the world continues to battle various mutations of COVID-19 virus, researchers are still looking for more accurate ways to diagnose and differentiate them from other diseases in a time-efficient way. For this purpose, they employ artificial intelligence and deep learning methods, which enable a quick and efficient analysis, effective comparison of radiological images as well as identification of changes in the lungs.
"It is important to mention that accurate interpretation of radiological image analysis and disease identification in the early stages are crucial as other tests, such as serological tests (antibody detection), can only confirm or deny the presence of the disease, which might get unnoticed due to virus mutations. They also cannot determine lung obstruction (narrowing or blockage of the airways) or other changes. However, radiological images processed by machine learning methods can accurately classify images and assign them to the corresponding disease," explains the researcher.
Text prepared by Milda Mockunaite-Vitkiene, Internal Communication Projects Manager at Public Communication Department, VILNIUS TECH.
Edita Mazoniene, a doctoral student at Vilnius Gediminas Technical University (VILNIUS TECH) Faculty of Fundamental Sciences, explores how artificial intelligence can assist in identifying the consequences of lung diseases in the early stages of diagnosis.
Treatment for lung diseases costs 380 billion euros annually
Over the past three decades, researchers when analysing various indicators related to chronic respiratory diseases and patient data have found that the prevalence of various lung diseases has increased significantly due to population growth, environmental hazards, and longer life expectancy. It has also been estimated that 4 million people worldwide die from lung diseases, and European Union (EU) countries spend about 380 billion euros on their treatment. Therefore, early diagnosis and accurate classification of the disease, detecting early changes, and assessing the cause rather than the consequence, could gave a great impact on progression of lung diseases globally.
According to E. Mazoniene, most cases of lung diseases go unnoticed. For example, tuberculosis in its early stages is often confused with other diseases, resulting in patients not receiving timely and appropriate treatment, further complicating the condition and progression of the disease.
For faster and more accurate diagnosis of such diseases, E. Mazoniene employs artificial intelligence in her research, specifically convolutional neural networks used for image recognition and object identification in X-ray image analysis.
"Convolutional neural networks can be trained to compare and properly classify radiological images by applying a deep convolutional neural network model that automatically identifies, for example, cases of tuberculosis. After multiple experiments, researchers have achieved an accuracy of up to 98.6%," explains E. Mazoniene.
These networks can also be used for lung segmentation, playing a crucial role in computerized diagnostics. This is because the entire set of acquired images is transformed into a dataset and then analysed internally, layer by layer, rather than visually. This approach provides reliable indicators, unlike decisions based on X-ray interpretations, where changes or forming structures are not always clearly visible, which then gets analysed by a radiologist. Therefore, such data analysis can become a suitable biomedical tool for early lung disease diagnostics, providing significant assistance to doctors in differentiating diseases or identifying early disease.
It will also help in the fight against COVID-19
E. Mazoniene explains that as the world continues to battle various mutations of COVID-19 virus, researchers are still looking for more accurate ways to diagnose and differentiate them from other diseases in a time-efficient way. For this purpose, they employ artificial intelligence and deep learning methods, which enable a quick and efficient analysis, effective comparison of radiological images as well as identification of changes in the lungs.
"It is important to mention that accurate interpretation of radiological image analysis and disease identification in the early stages are crucial as other tests, such as serological tests (antibody detection), can only confirm or deny the presence of the disease, which might get unnoticed due to virus mutations. They also cannot determine lung obstruction (narrowing or blockage of the airways) or other changes. However, radiological images processed by machine learning methods can accurately classify images and assign them to the corresponding disease," explains the researcher.
Text prepared by Milda Mockunaite-Vitkiene, Internal Communication Projects Manager at Public Communication Department, VILNIUS TECH.
Photos by Aleksas Jaunius.